5 Machine Learning Questions Answered
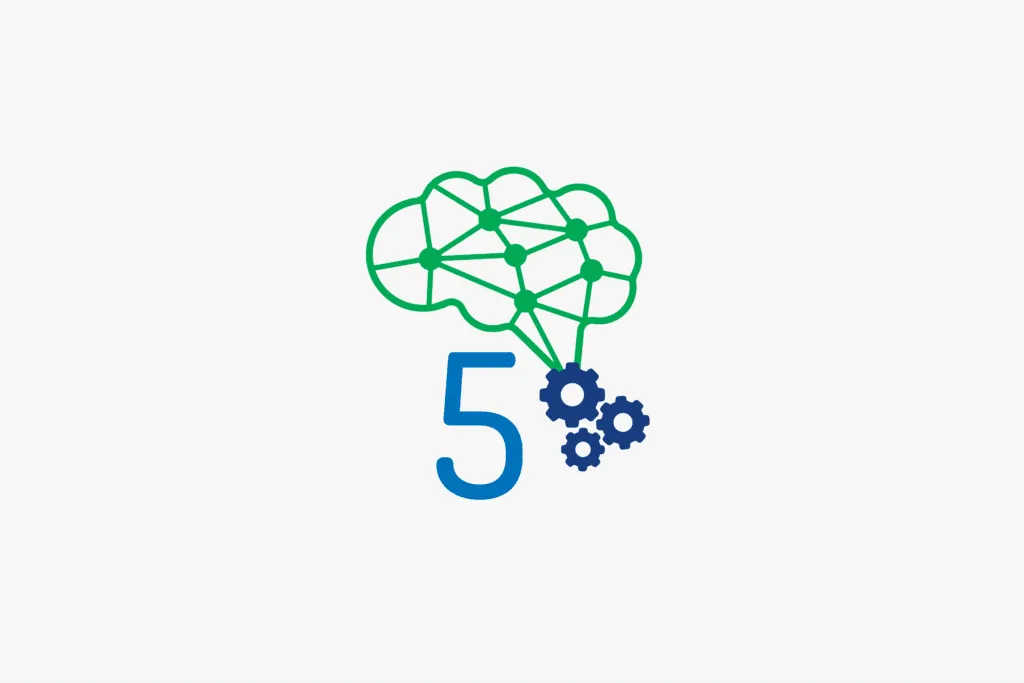
The concepts of machine learning aren’t necessarily easy for all of us to understand, but as the impact of AI technologies in life and work is beginning to be felt more keenly than ever, we have more questions about the difference they are making to our world. The continuous improvement in the speed of computing and our access to the vastly increased amounts of available data means that today, machine-learning applications sit in connected speakers in our kitchens and the phones in the palm of our hand.
What Do Machines Excel at That People Don’t?
Analyzing data to learn what’s actually happening and see trends is nothing new. To understand where machines are bringing an advantage in this area, we can look back at one of the earliest stories of data analysis. In 1854, London is in the grip of a cholera epidemic. At the time, it was assumed that cholera was airborne, but physician John Snow did not accept this “miasma” (bad air) theory, arguing that in fact, it entered the body through the mouth. To understand how the disease was being spread, Snow began to plot individual infections onto a map. This process revealed that a water pump in Broad Street was the source of the disease. John Snow had made connections in three sources of data (the map, the infections, and the water pump) to identify a trend and draw a conclusion.
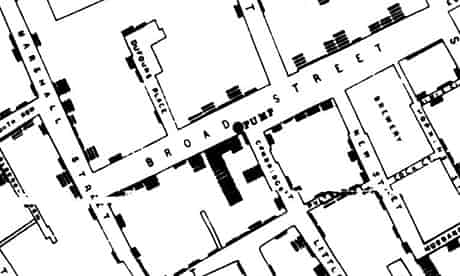
John Snow’s cholera map showing the pump on Broad Street.
Snow’s limited data set made his task the kind of focused process that humans excel at. Where computers have an advantage is accurately applying a process like this to huge volumes of data from numerous sources, at massive scale. The outcome of cross referencing from different sources enables the machine to create more relationships between the data at much greater speed than a human ever could.
Machines Can’t Really Predict the Future, Can They?
This question actually relates directly to the first one. Machines aren’t an AI crystal ball that can tell us exactly what will happen next week, next month, or next year, but because of the volume of available data, they can process and identify the emerging trends. We believe a better way to define this is the application of “predictive analytics.” This allows us to combine the historical data we see and the outcomes we’ve reached to make a reasoned assumption about what might happen in the future.
You can learn more about how predictive analytics are being applied to the field of cybersecurity in our latest white paper, “4 Ways Machine Learning Is Powering Smarter Threat Intelligence.”
To What Extent Can Machines Really Understand the World?
“Understand” is a difficult word to apply here. How intelligent or conscious a machine can be has frequently been the fodder of science fiction literature and cinema. The dictionary definition of AI is, “Giving machines the ability to seem like they have human intelligence.” One trait of intelligence would certainly be the capability to recognize objects appearing in particular images (which machines do quite well), or knowing the meanings of words and learning how sentences are constructed.
In the world of threat intelligence, our machine is able to do exactly this. Natural language processing means the machine can read and identify words in a sentence and understand how they relate to one another, and the more sentences it reads, the more it learns about how words are used, and in what context.
The difference between what a machine knows about the world and what we know really boils down to how we gather that knowledge. Some of our knowledge comes from what we see and experience, and some comes from data we gather and what we learn. The machine can’t experience things — it can only really gather the data and make relationships in the data it processes. But this does allow machines to be able to classify data, so they can learn that, “Paris is a city in France,” or, “Locky is ransomware used by the Necurs botnet.”
Should We Worry About Machines Stealing Our Jobs?
Today, the most powerful applications of AI and machine learning are when humans and machines work together to make use of both their strengths.
There’s no question that the nature of jobs is going to change dramatically in the coming years. The World Economic Forum expects automation and AI to result in the loss of at least 5 million jobs globally by 2020. Machines’ ability to process huge volumes of data and complete tasks in a more efficient way than a human being presents significant time and cost savings for businesses. It’s not an overstatement to say that we are moving toward a revolution in the way we work.
The advent of AI in the workplace will create roles we might find difficult to even imagine today, much as the internet’s arrival saw the birth of a whole range of new careers. Nobody’s grandfather was a web architect, but today, it’s a career with an attractive salary to match. It doesn’t matter whether a job is white collar or manual — if its routine, machines can do it.
Almost all industries will undergo a radical shift, so importance will be placed on acquiring new skills quickly. We’re unlikely to see mass unemployment, but as we’ve seen from technological progress in the past, businesses and employees must be ready and willing to skill up and retrain where necessary.
What Developments Will AI See in the Next 5 Years?
AI now feels a little like computing did back in the 80s. PCs were beginning to show businesses beyond just data processing companies that computers had real value to bring. Many more companies were creating computer divisions. Those divisions are long gone, with computing having become a ubiquitous part of our work, not to mention our everyday lives. AI is being, and will continue to be, quietly adopted by enterprises, allowing them to extract knowledge from all the data that is being generated — and not just the structured data.
The aim for many enterprises in the short to medium term will be to move AI systems to take on decision-making tasks like managing inventories or screening candidate resumes. These jobs are time consuming for humans and so present a significant cost saving for businesses. However, despite the rapid adoption of domestic assistants like Google Home and Amazon Alexa, consumers may prove a harder proposition, particularly in the much vaunted area of self-driving vehicles. Recent research from MIT found that while people have become more accepting of driver assistance features like automatic emergency braking and blind-spot warning, doubts about fully self-driving cars are growing. This is a clear indication that the applications for AI and machine learning still need to meet a demand and deliver a tangible benefit to experience widespread adoption.
Related