Dark Networks: Social Network Analysis of Dark Web Communities
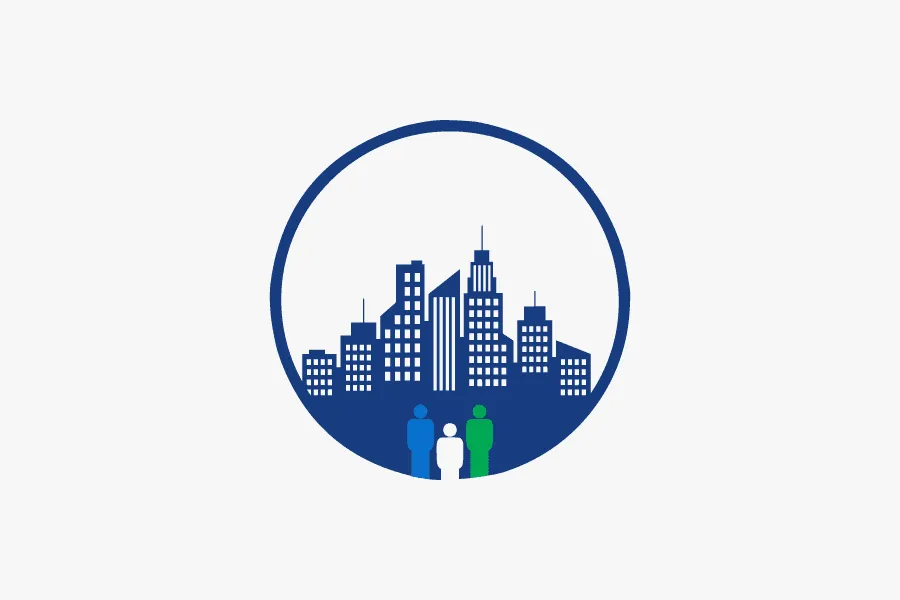
Key Judgements
- We found that the dark web is organized in three distinct communities: low-tier underground forums, higher-tier dark web forums, and dark web markets.
- We observed that there is a significant group of actors posting in both low-tier and higher-tier forums, showing that there is a connection between these two communities while the markets are almost completely disconnected from them.
- By using the centaur approach of combining human and computer intelligence, we organized our collection of dark web and special-access sources into categories that reflect our findings.
Executive Summary
Not all dark web data is the same. As a result of our thorough collection of dark web and special-access sites, we ended up with hundreds of sources in one uncategorized pile. The value of the data obtained from these sources varies depending on each user’s intelligence needs, so we needed a more granular source classification.
The main challenge of a more granular classification is the amount of sources — there are just too many to categorize manually one by one. We turned to the practice of social network analysis of authors to solve this problem. This data analysis technique allowed us to build our dark web source categorization from data-driven suggestions instead of a blank slate, using the centaur approach to take advantage of both human thinking and AI-enabled technology.
We found three distinct communities of actors in dark web and special-access sites: low-tier underground forums, higher-tier dark web forums, and dark web markets. These three clusters line up with our expert intuition of the dark web, appearing almost as if no other sensible organization is possible in retrospect. Additionally we found notable cross-posting between low-tier and higher-tier forums. The results of this research are directly reflected in Recorded Future’s product and ontology. This new categorization helps security teams obtain targeted, relevant dark web intelligence, facilitates their understanding of threats, and opens a window into the methods, tactics, and motivations of threat actors.
Methodology
Our main goal for this research was to organize our dark web sources for analysis. We used social network data mining techniques, a widely adopted approach that focuses on the structure of relationships within a set of actors. Our analysis led us to patterns within the data that allow us to understand the character of different segments of the dark web social network.
For this investigation, we relied on Recorded Future’s collection of dark web and special-access sites, focusing on threat actors that owned multiple user accounts across many dark web sources. This data is based on the assumption that individuals use the same handle across forums. We consider this hypothesis to be reasonable, due to the fact that identity, reputation, and credibility are as important to threat actors as it is to typical internet users.
Based on the full post history of the selected dark web members, we constructed a weighted, undirected graph comprised of a set of around 1,000 vertices joined by more than 200,000 edges. Each vertex represents a threat actor. The weight of each edge is proportional to the number of sources where the two threat actors that are the endpoints of the edge are both seen as an author. The social network analysis relied on the edge weights, and in addition, we retained for later analysis the list of specific sources represented by each edge.
Figure showing the relationship between nodes and edges. Node “X” is connected to nodes “Y” and “Z” through edges “i” and “j.” Each edge has an associated weight (M, N) corresponding to the number of common sources between two threat actors (i.e., Source 1, Source 2, ..., Source “M”).
Working with social networks is challenging, especially when it comes to visualizing the data. The first representation of a network can often result in a “hairball,” meaning a confusing plot of overlapping edges and communities with no analytical value. To avoid this, our first analytical step was to generate a standard force-directed layout based on edge weight. Force-directed graph drawing is a widespread technique that assigns forces among the nodes and edges of a network. In our case, this technique will pull threat actors with many shared sources close together and push threat actors with few shared sources apart.
Visualization of the threat actor network using a force-directed layout algorithm.
The first visualization of our network showed an emerging structure of nodes into subgroups. This information suggests that one feasible way of organizing this data is through clustering, motivating the question of how many communities we could obtain by analyzing the relationships within threat actors. To achieve this goal, we used modularity, a measure of the structure of networks designed to gauge the quality of the division of a network into modules. A good subdivision of a network has high modularity; within modules, the nodes have dense connections, but these connections become sparse between nodes in different modules. We used the Louvain method, an algorithm capable of handling large, weighted networks in a short computation time with excellent accuracy, to calculate the modularity of our network.
Side-by-side comparison of two visualizations of a network representing the relationships between the characters of the novel Les Misérables. On the left, the network is displayed without any metrics. On the right, we represent the results of running the Louvain method on the network, rendering six communities here represented in different colors. As we can observe, the analytical value of the network increases, showing relationships that were not obvious at the beginning. Credit: Network data obtained from the Stanford GraphBase, 1993 Stanford University.
Result: 3 Dark Web Communities
The Louvain algorithm returned three main clusters:
Plot of the threat actor network showing the three main clusters with a modularity-based layout. The nodes are color coded depending on what cluster they belong to. Nodes that do not belong to any clusters are represented in grey. The most connected nodes are attracted to the center of their clusters.
What is the difference between these three clusters?
By examining the sources previously tagged on the edges of our network, we can determine what sites are the most common among actors that belong to a cluster. For each of the three clusters, we used our expertise in dark web collection to assess the main sources within the cluster, and it became clear that the three clusters correspond to these communities:
- Low-Tier Underground Forums: Usually free and open-access forums, with many novice members.
- Higher-Tier Dark Web Forums: The access is generally restricted through things like strict membership vetting, only hosting the site on Tor, or other requirements for access. Members of these sites are experienced and regarded as reputable by other members of the criminal community. Rippers (members that scam other members without delivering a good or service) are scarce, and rigorous banning is enforced in order to protect the community.
- Dark Web Markets: Market sites with listings of illicit services and goods, stolen credentials, credit card dumps, etc. The access is usually open, meaning that they do not require an existing member to vouch for new registrants.
The presence of edges between the two forum clusters versus the almost complete disconnection of the market cluster shows that there is a greater division between forums and markets than there is between low-tier and higher-tier forums. There is a significant group of actors posting in both low-tier and higher-tier forums, while the actors that frequent markets do not contribute in the other communities with the same usernames nearly as often.
One possible theory about this pattern is that threat actors in higher-tier forums frequently visit and contribute to lower-tier forums to identify trending topics and build reputation. Dark web market users, on the other hand, may not be interested in the topics discussed in the other two communities, or they could be using different personas to further protect their identities.
After final analyst validation, we organized our ontologies to reflect these findings. They are visible in the Recorded Future user interface as three categories within our source types: Forum – Underground, Dark Web / Special Access Forums, and Dark Web Markets.
Conclusion
We used social network analysis on threat actor data to reveal that the dark web is organized in three distinct communities: low-tier underground forums, higher-tier dark web forums, and dark web markets. Recorded Future’s dark web source organization reflects these communities, allowing our users to combine targeted, relevant information from the dark web with technical and open sources to obtain valuable threat intelligence.
To learn more about how you can get valuable threat intelligence from dark web sources, request a personalized demo.
Related